

Consistent involvement of data management personnel in analysis planning and dataset creation can also provide an opportunity for standardization of code and processes. Data management personnel responsible for preparing the data for analysis will derive insight from an understanding of the objectives for modeling and simulation efforts, appreciation for the types of data that are most critical to meeting analysis assumptions, and in addition, can provide insight and refinement of data editing rules. Team members representing various functional areas can provide differing types of input and derive different kinds of benefits from involvement in the analysis planning process. The benefit of project team alignment prior to analysis and agreement with the planned approach to support eventual claims based on modeling efforts will only serve to further ingrain and instantiate the use of modeling and simulation techniques as an essential critical path activity. Besides the improvement in analysis quality that derives from such careful a priori planning, obtaining input and/or approval of a pharmacometric analysis plan from team members representing other disciplines will likely result in greater buy-in and support for analysis results. The mere act of prespecifying, in detail, the major analysis steps and contingencies often results in a discovery of some sort: a feature of the data requiring further processing for analysis, an exploratory plot that might provide additional insight prior to modeling, a modeling assumption not likely to be met, a data editing rule requiring refinement, or an additional model worthy of consideration. The two most relevant regulatory guidance documents for population PK/PD modelers ( FDA Guidance for Industry, Population Pharmacokinetics (Food and Drug Administration 1999) and EMEA CHMP Guideline on Reporting the Results of Population Pharmacokinetic Analyses (EMA 2007)) both refer to the importance of analysis planning by stressing the need to specify analysis assumptions and decision-making rules, as well as deviations from the planned analyses. International Conference on Harmonization (ICH) guidelines on Statistical Principles for Clinical Trials (European Medicines Agency (EMA) 1998) recommend finalization of the SAP before the study blind is broken, in addition to documentation of such activities.

A well-written SAP should contain enough detail to enable two independent analysts following the plan to come to essentially the same conclusions.
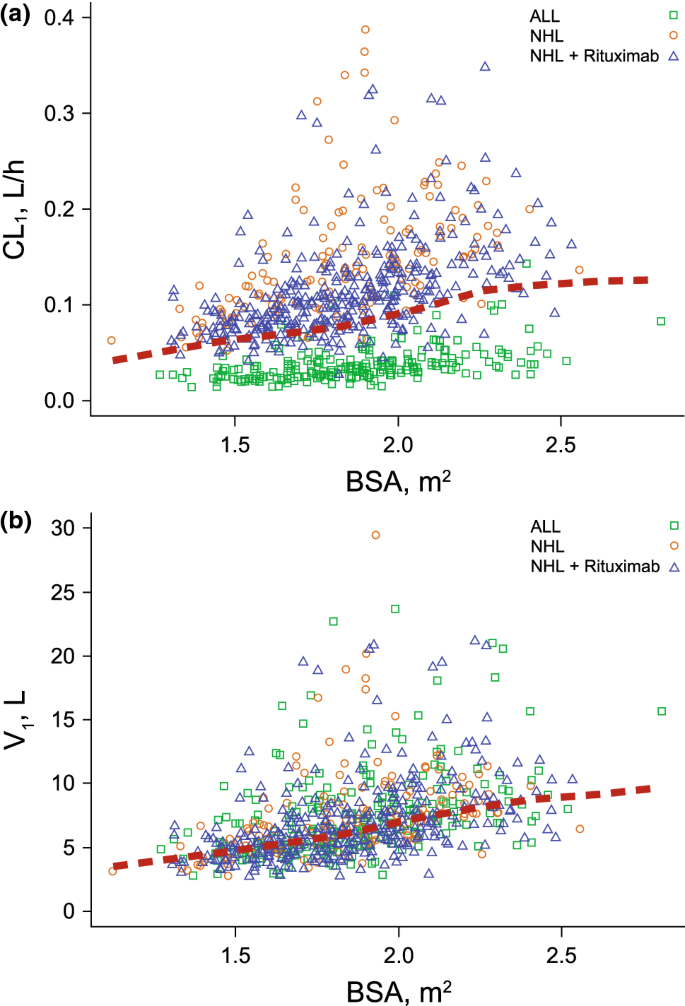
Development of an analysis plan prior to locking the study databases, unblinding the treatment codes, and/or analysis commencement is perceived to be good statistical practice to minimize potential bias and lend credibility to analysis findings. Statistical analysis plans (SAPs) have long been used by statisticians in various fields of study to promote the ethical treatment of data and to ensure the consistency and repeatability of analyses. Whether the analysis planning process entails the development of a formal, controlled, and approved document or simply a bullet-point list describing the main components of the plan, it is critical to take the time to complete this step prior to initiating data analysis. Today, however, many modelers have adopted their own unique variations on this framework in terms of the extent to which process steps are completed and the order in which some steps are undertaken.Īnalysis planning is perhaps the most important step in any data analysis and modeling effort but particularly so in pharmacometric model development and analysis. Ette and colleagues extended this process beyond modeling to address steps that should be taken in advance of modeling and steps that may be taken after a final model is selected to address the application of the model to the problem at hand (Ette et al. A high-level description of the proposed process is illustrated in Figure 5.1 each of the steps outlined in the figure will be discussed in this or subsequent chapters of this text.įigure 5.1 Typical pharmacometric model building process.Ī framework consisting of the modeling-related steps (i.e., basic model, addition of structural features, addition of statistical features, model refinement, and model testing) was first proposed by Sheiner and Beal in the Introductory Workshops they offered in the early nineties, and became fairly standard practice amongst many pharmacometricians (Beal and Sheiner 1992). This framework consists of the basic steps of the process for the development of such models (in the context of their application in a drug development endeavor). Owing to the hierarchical nature of population pharmacokinetic/pharmacodynamic (PK/PD) nonlinear mixed effect models as well as the typical complexity of such model development efforts, a general framework is proposed.
